Big tech’s ‘Agentic AI’ gamble: can massive spending deliver?
As the new year begins, concerns grow over a top-heavy US stock market dominated by the Magnificent 7—Apple, Alphabet, Amazon, Meta, Microsoft, Nvidia, and Tesla.
Both the Mag 7 and investors have put eyewatering amounts of capital on the line — in the name of AI.
While the market has rewarded the Mag 7's AI initiatives so far, the real test lies in their ability to monetize these massive capital expenditures in the future.
This year, that monetization push will be ‘Agentic AI: your everything helper’. That’s AI that can not only understand your requests, but action them.
You can think of it as the difference between a helpful librarian who answers your questions and a personal assistant who not only answers questions but takes initiative, makes plans, and carries out complex tasks on your behalf.
For now, ‘Agentic AI’ remains a buzzword. But it could be the x-factor supercharging AI adoption in the coming year… or become the word on this market cycle’s epitaph.
Is this the Hail Mary pass to drive big tech higher?
The Magnificent 7 Arms Race
Without the Magnificent 7, the S&P 500 would have only gained 4.1% in 2023 and 6.3% in 2024. Instead, we’ve seen two years of 24.2% and 23.3% returns and unprecedented concentration in the major indices.
Whether a correction comes or not, it’s no secret that the market has been skittish about the group’s high valuations and spending.
Recent earnings from the Big Four (Amazon, Meta, Microsoft, and Google) have all seen huge volatility as they each announced increased Capex spending in the AI arms race.
You can already see (below) just how much their spending has ramped up in recent years:
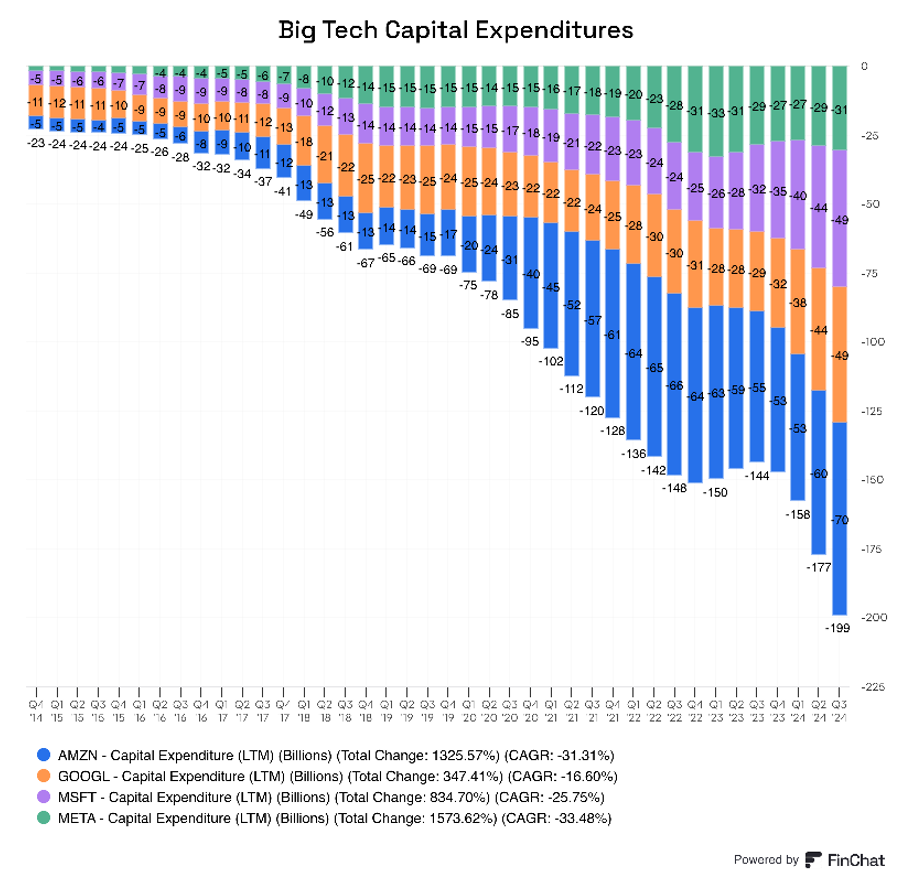
Like last quarter, all four have stuck to their plans to ramp up capital expenditure (Capex) on data centres and AI.
Looking at the third quarter compared to the same quarter last year:
- Amazon’s capex rose 81% to US$22.6 billion
- Alphabet’s capex rose 62% to US$13 billion.
- Microsoft’s capex rose 50% to US$14.92 billion, with a further US$5 billion in finance leases for data centres.
- Meta’s capex rose 36% to U$9.20 billion and said they plan to spend ‘significantly more’ in 2025.
According to Barrons, the combined capex of the largest US tech firms is forecast to rise 16.5% in 2025 from US$218 billion to US$254 billion.
While these companies don’t directly report where all that capex is going, we can surmise that most of this is going into AI Infrastructure.
That is, buying GPUs from Nvidia (which still controls around 82% of the market share), building and retrofitting property for data centres, and leasing land.
These land leases have become a huge part of their spending in the last two years. Microsoft, for example, has over US$100 billion in leases that have not commenced — what looks like a clear overspend.
Here’s what that lease ramp-up looks like for Microsoft:
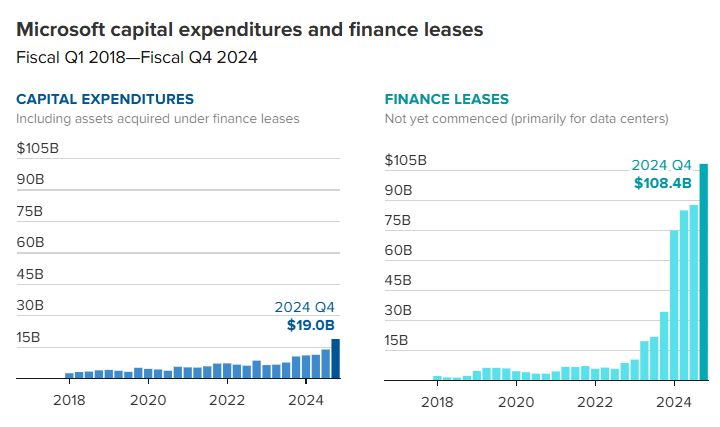
These leases are a mix of vacant land and existing data centres from providers like Oracle. Trying to parse the length and costs of each lease then becomes another challenge for investors to weigh.
Many involve 15-year leases and include large tax abatements that muddy the picture. If things don’t go as planned, these could weigh on future profitability. For now, they’re happy to hold the potential capacity in case things go parabolic.
Similar things are happening at the other giants who are in a mad dash to accumulate water, power, and land to fuel AI.
Some have criticised this spending, with sceptics arguing that the future revenue isn’t there to support such massive outlays of money.
In their last earnings call, Google’s CEO, Sundar Pichai, fielded several questions from concerned Wall Street analysts about the return on investment of all this capital.
Barclay’s analyst Ross Sandler noted that if this capex ramp-up continues, AI might go from an ‘underbuilt situation’ in 2023 to ‘potentially being overbuilt next year’.
Sundar Pichai’s response?
‘When you go through a curve like this, the risk of underinvesting is dramatically greater than the risk of overinvesting’
But how should individual investors think about these massive spends?
Not just in terms of the companies involved but the broader picture of AI investment opportunities before us.
A great critique of this capex race is the comparison to the dollar-cost auction. Simply put, it's where spending becomes irrational for fear of being a second-place loser who still has to pay.
They're caught in a competitive spiral where the fear of losing (market share, technological edge, talent) outweighs the massive costs they're incurring.
In the eyes of the tech giants, losing the AI arms race is existential.
While the dollar-cost critique has some merits, the key difference is that unlike the dollar auction (which has a clear negative outcome for both parties), the AI arms race could lay the foundations of a new tech future.
Yes, these massive companies could be overspending now. However, like Cisco in the dot-com era, the technology built will create a springboard for future growth.
But that doesn’t mean you wanted to own Cisco stock after the dot-com era. Could Nvidia follow the same trajectory?
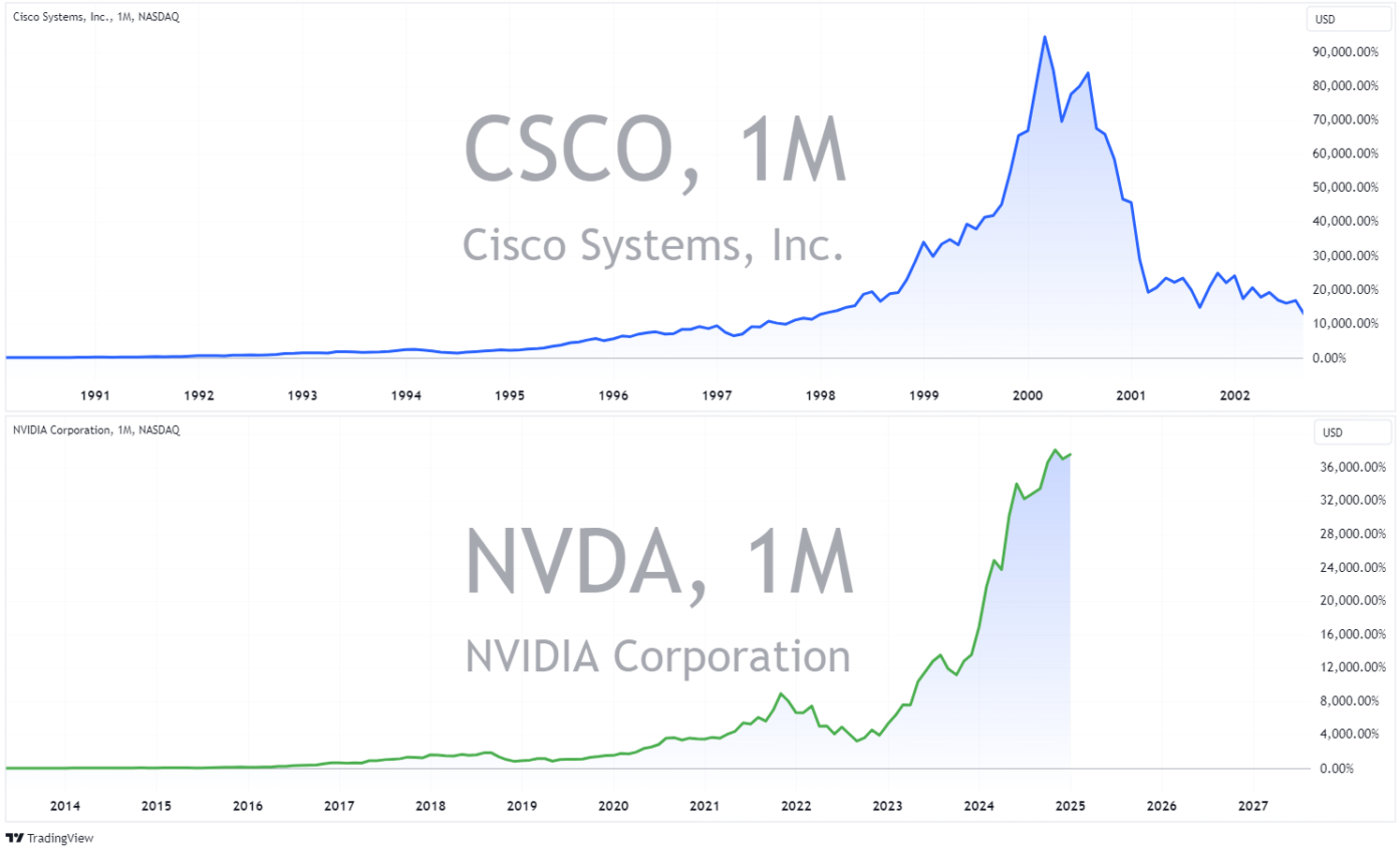
I think the comparison is unfair for now. Nvidia’s P/E is around 48x, while Cisco’s nearly touched 200x before the crash.
Plus, much of Cisco’s revenue came from builds in anticipation of internet growth, while Nvidia’s GPUs are being deployed today.
These GPUs also have a much shorter useful lifetime before they need to be replaced, which could cut down on the chances of overbuilding.
However, the rhetoric around Nvidia and Cisco is strikingly similar. Dominant tech stocks with 80%+ market share and near unlimited growth potential sounds a lot like market mania of the past.
So, how can AI market growth continue to scale?
In my opinion, AI's trajectory now hinges on how profoundly it transforms the workplace. This all depends on whether Agentic AI is the real deal.
First, let’s unpack the limitations of AI in the past few years to understand what makes it different from Agentic.
What’s Held AI Back
You’ve probably heard of Generative AI (GenAI). These are systems that use deep learning models to process vast amounts of data to generate new content.
However, the content isn’t exactly new; it's making connections between the information it has seen during training.
Breakthroughs in abstraction, pattern recognition, and contextual understanding combined with raw computing power create today's AI, which is impressive in itself.
But these AI models are severely limited. They think ‘inside the box’ and lack reasoning. This boils down to a highly statistical response to a series of past data.
This can work remarkably well for something like language, which is very linear. For example, If I said, ‘the quick brown fox jumps over the lazy ‘...’
Both you and the AI know the next word is dog. Both rely on memory in a way, even though many of you may have forgotten why it’s dog!
In this way, past examples can truncate or determine the outcome. A more visual example can be found in images that AI produce.
If you ask any AI to create a picture of a watch with a very specific time (say 5:30, as I requested below), you will get a response similar to this:
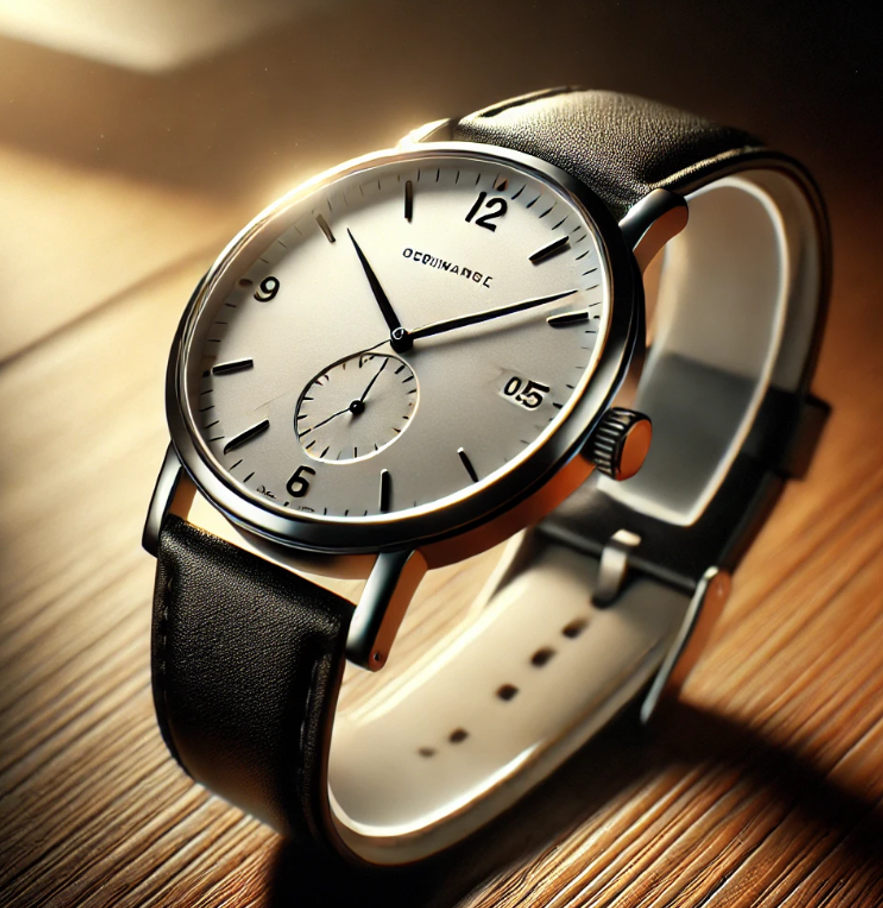
Why? Marketers decided many years ago that 10:10 was a beautiful way to display a watch. So, most examples that AI has seen in training display this time.
These GenAI lack the reasoning to connect ‘the time’ with the position of the hands. Instead, they rely on their past examples, so in the eyes of AI, this is what a watch must look like.
You can think of it as a student who memorises test answers without understanding the core concepts.
This has been the state of many LLMs and GenAI tools these past few years — parroting tools with limited scope to reflect, reason, and therefore meaningfully unlock productivity.
Because of this, robust, business-wide applications have yet to materialise beyond a Q&A and content generation machine.
But this is where things could change. This year, things are about to be very different. Let’s delve into the world of Agentic AI.
The Agent for Everything
Just when you’ve wrapped your head around a concept like Generative AI or Large Language Models (LLMs), Silicon Valley throws a new one at us.
Agentic AI: The AI agent that could assist you in everyday tasks (or potentially replace you).
Microsoft was the first to attempt an all-encompassing AI helper with Copilot. So far, it’s seen a lukewarm reception.
Salesforce’s CEO Marc Benioff recently threw jabs at Microsoft’s Copilot, claiming it was akin to ‘Clippy 2.0’, a callback to the annoying 90s Word assistant.
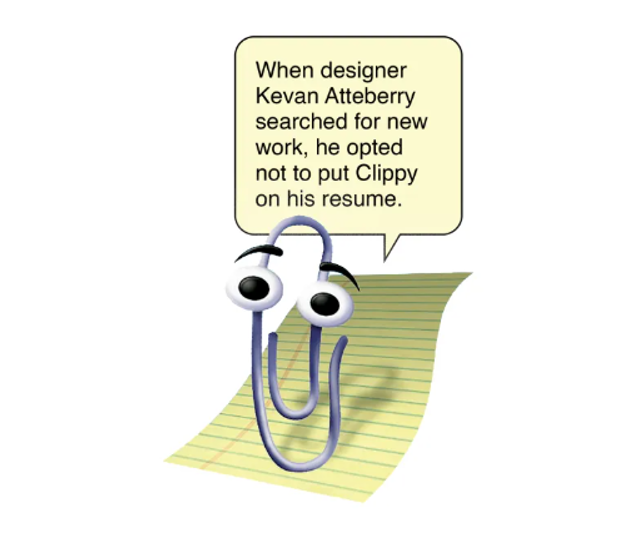
I will admit to testing Copilot the least of all the major AI. After some early 2023 testing, I quickly gave up after a series of poor answers and limited scope for action.
But things move quickly in AI, and 2025 is slated to be the year of change. A year where AI will evolve from ‘that clever chatbot’ to AI that can think ahead and help you achieve specific goals.
This hasn’t come from a single major breakthrough but from combining many teams' small gains.
This rate of progress has seen the inference costs (cost to run AI requests) fall by 99% in the past couple of years and a steady improvement in reasoning benchmarks.
Open AI's recent O3 models are also worth a look. The old paradigm of 'more data' + 'more compute' creating the best AI is now being challenged by 'more time thinking' + 'more compute'.
We still don't know how far that rabbit hole will go, but it has vast implications for the economics of 'cheap AI for all' when these new models can cost $30-3500 per request.
But what about the intelligence of the Magnificent 7 AI?
In December, Google released its latest AI called ‘Gemini 2.0’, calling it ‘our new AI model for the Agentic era’. Looking past the marketing fluff, the major changes that define it are:
1. Multi-modal interaction and memory: AI now has the tools to see, hear, and interact across many domains. It can understand a video, photo, website, or real object. From there, it can interact with your phone, web browser, or robotics.
2. ‘Chain-of-thought’ reasoning: The race for AI to respond quickly and cheaply drove research into a wall. New progress has given AI the early stages of pseudo-reasoning. This can help it think through a task step-by-step and refine its decision-making process.
3. Goal-oriented algorithms: Systems are designed to work towards specific goals rather than respond to a question. This is achieved through ‘Agentic Frameworks’. You can think of them like a Matryoshka doll-like structure of nested AI, each with its own function working towards the greater task.
Putting all this together, you have the beginnings of systems that can think, plan, remember, adapt, and take appropriate action.
Okay, so that’s the gist. Now, I can understand you’re probably sceptical.
We all remember buzzwords like ‘Blockchain’ or ‘Web 3.0’. These words are now relics. Why won’t Agentic be another?
Because, unlike those words of yesteryear, Agentic represents something that will actually have real-world use.
Adoption curve
At the beginning of 2024, the adoption of AI had already begun to ramp up.
Around 72% of organisations had already incorporated AI into one business function, while over half had multiple functions AI was helping with.

It’s of little surprise that most of that early AI use is supporting marketing teams with content. At this stage, companies are happy for AI to be another low-level content writer.
But this new era promises personal assistants for each and every one of us.
Imagine everyone on your team having their own PA who can look at your calendar, make proactive recommendations, and take action on your behalf.
Or meeting minutes that are automatically transcribed and sent to relevant parties in their native languages with individualised responsibilities, dates and actions to take — many of which are already begun by the AI and waiting for your approval.
The possibilities from here are vast and will eventually be fine-tuned for almost every sector or job.
In healthcare, for example, Agentic AI accelerates drug discovery by autonomously designing and running experiments at companies like AstraZeneca, Pfizer and Recursion Pharmaceuticals.
The Mayo Clinic is currently piloting AI that analyses patient data, recommends treatments and coordinates follow-ups.
Of course, these examples are limited. We are still early in this new era. But once the issues are ironed out, adoption will explode.
Things are already moving quickly. Generative AI has a 40% adoption rate in just two years—twice as fast as the Internet or personal computers.

With that comes the demand for the companies powering it — data centres, energy companies, and many of the Mag 7.
Looking at the bigger picture, we saw in 2024 that businesses were reluctant to shift company-wide systems towards AI.
Many were waiting until they felt the outcomes were clearer and their brittle systems could handle it.
Many of these legacy constraints will remain for a time. Siloed and poorly structured data and workflows are rife in large businesses. Plus, traditional IT governance and security struggle with AI’s need for broad data access.
But a key thing about AI you need to understand... Its impact won’t be incremental. Like the internet, it’ll result in massive business model re-designs that completely upend old models.
I would argue that this next era of Agentic AI will tip that major shift. The Mag 7 certainly thinks so. They are laying their fortunes on a future in which these AI will define the workplace of tomorrow.
To return to the question, are the Magnificent 7 likely overspending on AI right now? I believe the answer is probably. But that doesn’t mean there isn’t opportunity for investors right now.
If large enterprises want any hope to adopt AI, they will need to invest heavily in their data and governance — find companies that assist the transition.
Or, look for sectors where the major players are lagging behind in tech. Invest in smaller, nimbler companies that could overshoot the relics of the past with the help of AI.
The risk of a correction for the Magnificent 7 is real, but who knows when? If that occurs, then real value buying opportunities will be hard to ignore.
For those who hold them now, be aggressive with your stop-losses if you’re concerned about the short term, and don’t sweat it if you believe in the long term.
Of course, facts are changing quickly. The speed of AI’s advancements has made predictions about the future more tenuous than ever. Keep an open mind.
For those who’ve used AI in the past for work but were disappointed and forgot about it — give it a try now.
Then, mark this moment and see where they are by the end of 2025.
If you want to read more articles like this, sign up at Fat Tail Investment Research to see more market-leading topics on AI, gold, commodities, and macro trends.
%20cropped.png)
2 topics
3 stocks mentioned