Move over God-Like AI, here comes Larry!
Some innovators were legends before anybody knew, but them. This is the stuff of crash through, or crash, confidence. The kind that can make, or break, a firm. The industry legends that surround Oracle Executive Chairman, and Chief Technology Officer, Larry Ellison, are many, but none equal to the confidence expressed by the man himself.
To be fair, much of this ego-spritz is warranted.
Oracle has made its share of mistakes, like the Sun Microsystems acquisition, but the firm remains a powerhouse in database management systems and has successfully navigated towards a cloud-based Software-as-a-Service (SaaS) business model.
Our interest is the next phase of Oracle business development, which leverages their customer base in data management to the next level with Infrastructure-as-a-Service (IaaS), built on the Oracle Cloud Infrastructure (OCI) product.
This is the AI Cloud or Cloud 2.
The firm appears to be in the sweet spot of a major turnaround with an AI tailwind.
The history behind SQL the language and Ellison the innovator
While younger technology investors may not think much of the Structured Query Language (SQL) database, this technology still lies at the core of most corporate data strategy. What most folks likely forget is that Larry Ellison co-founded Oracle as "Software Development Laboratories" way back in 1977, to put into practice Edgar F. Codd's revolutionary idea.
Codd worked out the mathematical theory of the relational database way back in 1970 when working for the then-dominant computer company IBM. That firm dithered with the idea, in the common pattern of technology incumbents, which opened the door for Ellison to be among the first entrepreneurs to fully capitalize on implementing the technology.
The opportunity slipstream that IBM handed to Oracle, and others such as Ingres, Informix and Sybase, followed by Microsoft, was the UNIX and Windows PC market. IBM dominated mainframe hardware and used DB2 and SQL/DS sales to help move hardware.
Ellison and his co-founders had the vision to chase down the non-mainframe market that was then emerging around the UNIX operating system, and the IBM-compatible PC market. This ancient history is made fresh again, by the emergence of generative artificial intelligence.
How so, you might ask?
Well, it all comes down to the refresh of big iron, or scale-up computing, as we now see with the ascendance of NVIDIA selling the world on the benefits of accelerated computing.
This story is likely to unfold visibly in the coming competition between incumbent hyper-scale cloud operators, such as the big three Amazon AWS, Microsoft Azure and Google Cloud, a host of start-up niche players, such as privately held Lambda Labs, Paperspace, and VastAI, along with cloud latecomers, such as IBM Cloud, Tencent Cloud and Oracle Cloud Infrastructure.
While we own Microsoft NASDAQ:MSFT for exposure to the main hyper-scale AI theme, chiefly due to their long association with OpenAI, and the preparatory work done by that company, we just added a position in Oracle NASDAQ:ORCL, for reasons I will now elaborate on.
In a nutshell, we like Oracle, in spite of their clear "late entrant" status because their database heritage gave them the confidence to bet large, in a timely fashion, on hyperscale for the vitally important high-performance networking infrastructure to run deep learning, at scale.
If you want to understand more and learn why Oracle Cloud Infrastructure is on speed dial for a host of new well-funded generative artificial intelligence start-ups, then read on.
The Network is the Computer™
Sun Microsystems is a defunct high-end server company, now owned by Oracle. I remember making ten times my investment in SUNW, back in the 1990s tech boom. Here is the trade, number one, for my partner and I (who co-owns and co-runs Jevons Global), in the USA.
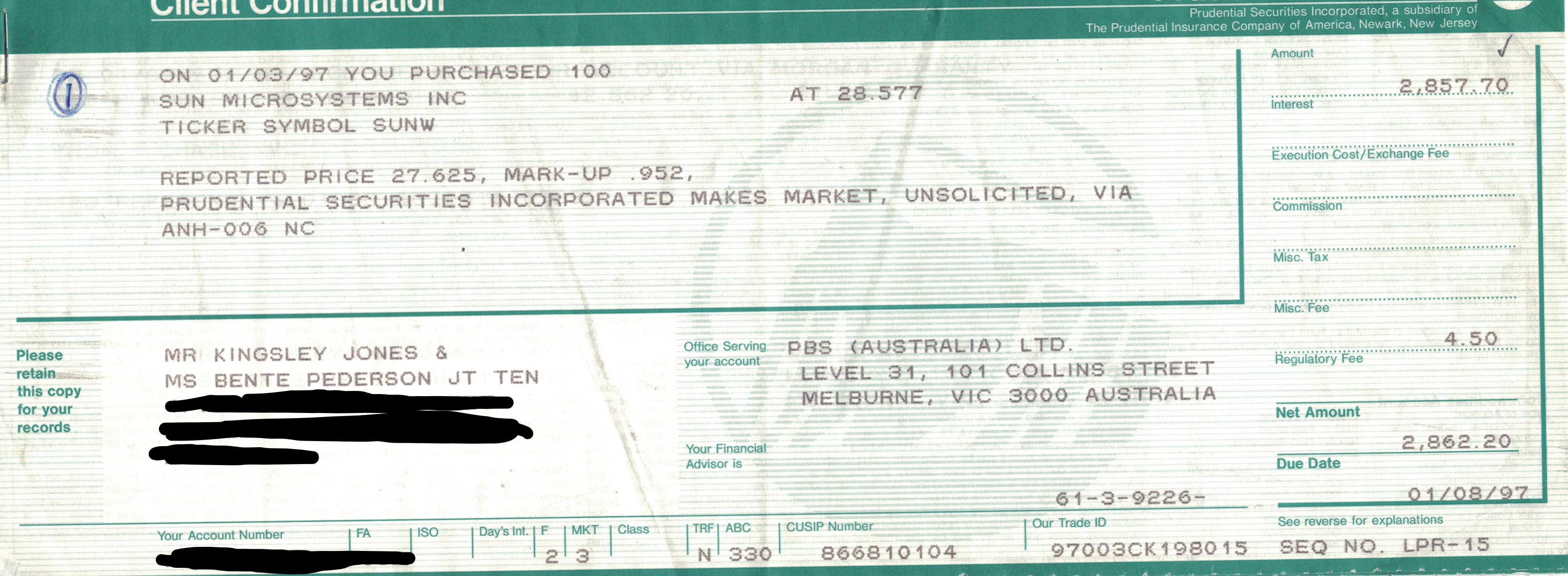
This trade is worth sharing for several reasons, not only personal nostalgia. Firstly, then as now, there was very little Australian press coverage of global opportunities. You really had to do your own research. Secondly, then, unlike now, brokerage was expensive. This trade cost over one hundred dollars to execute. However, when we sold in 2000, this came down to $40 on a parcel that was around ten times larger in value. Finally, then, as now, it was very hard to find an Australian accountant who could do foreign stock tax reporting. That part has not really changed at all, so we taught ourselves to do it, and then taught that to our accountant.
In those days, I worked in Australian Defense R&D, as a Research Scientist at DSTO. Every day my colleagues and I worked with high-performance computers for military applications. This generally entailed having two computers: a low-powered Windows PC for Excel, and a UNIX workstation from Sun Microsystems, or Silicon Graphics (SGI), for anything serious.
My partner was a business manager at a software firm in St Kilda, Melbourne, that specialized in staff rostering software for a range of verticals from airlines, through hospitals to casinos. Their software was compute-intensive, due to the nature of the scheduling optimization, and needed to also access a corporate database, almost always Oracle. The result was that clients of her firm almost exclusively purchased Sun Microsystems servers to handle the workload.
This period was great, for both Oracle and Sun Microsystems, and each boomed. The internet was growing rapidly, but search engines were barely monetized, and most ecommerce sites were still ramping up. Check out WayBack Machine for the Amazon website in July 2000.
Current gamers will be pleased to hear that, way back then, "Diablo is back!". That was Diablo 2!
Sun was giving a good business generation shout-out to "Dot-com Heroes!".
NVIDIA had just released their GeForce 2 graphics gaming card for Windows PCs.
Oracle was deeply embedded in selling Larry Ellison's vision of B2B Internet business.
Google was still privately held but had just signed up Yahoo as a search engine customer.
Needless to say, my partner and I did well out of that SUNW trade. However, we none of us then knew quite how the rise of Google would affect the economics of computing.
The tagline "The Network is the Computer" was a Sun Microsystems rallying cry, which we all thought made perfect sense at the dawn of the Internet Age. However, what many in industry and the market were not prepared for, was the rise of commodity computers.
To properly understand today, we need to know why that made perfect sense as the key next step for monetizing the Internet, at scale, with the birth of scale-out cloud hyperscalers.
Don't Scale Up - Just Scale Out!
Sun Microsystems struggled through the 2000's Internet bust with a free software platform called Java™ that won accolades, but generated little revenue, and a high-end UNIX server hardware business, that increasingly sold only into corporate SQL database applications.
The commodity x86 market of Intel chips NASDAQ:INTC, and later NASDAQ:AMD, took over.
What radically changed the market was a happy circumstance for search engine vendors, such as Google. The type of algorithms that were needed to build a search index, and also to serve results from that index, were capable of running well across many lower-end computers.
Google was among the first firms to recognize that a major monetizable workload could be run very efficiently on loosely networked commodity hardware, including disk drives.
This was a visionary move, on their part, since they needed to do the necessary development to ensure that a vast number of interconnected commodity computers could deliver the type of reliability needed for the search query application. Part of this effort consisted of building a very reliable file storage system, the Google File System, from many unreliable parts.
This idea, in many forms, has repeated itself throughout the history of computing. It goes back to the very early work of computing pioneer John von Neumann. He wrote a paper in 1952 that took up the question of how natural systems use redundancy and error correction to make up for naturally faulty hardware. That paper mentions probability logics, which lie at the heart of modern generative artificial intelligence. However, in the case of Google, the key idea was simply redundancy, storing many copies of the data, with suitable corrective software.
This approach worked extremely well and could cope with low-end networking equipment such as commodity Ethernet cards, routers and switches. In simple terms, you could go to BestBuy, run up the card for a box of parts, and go build a cloud supercomputer.
There are several reasons why this worked very well for the cloud era. Firstly, the search engine algorithms, like PageRank, could run effectively over such commodity clusters. Secondly, the nature of web traffic is largely uncorrelated. In effect, the customer interactions are going to be independent, and so there is no need to share any data between them. Finally, the network traffic can be served by aggregating many thin network pipes into one fat network pipe.
Just to make this clearer, what Google figured out is that their kind of supercomputer, one that fitted the cloud era of shopping and online advertising, really did not need the expensive and hard-to-configure networks and servers of the Sun Microsystems era.
For full context, Marc Andreesen, the original architect of the Mosaic browser, which led into the runaway IPO of Netscape Communications wrote the original code at the University of Illinois Urbana-Champaign National Centre for Supercomputing Applications (NCSA).
As often happens with innovation, the kind that was needed to move forward, was not that practiced in the place that Andreesen learned his trade. The sort that they did at the NCSA involved hundreds, or thousands, of high-end computers, networked into a supercomputer that did a form of computation that required high levels of communication between all computers involved. This is the tradition that Google broke with, because it worked for them, and for the problem they needed to solve, at that time, twenty plus years ago.
We call this the Cloud 1.0 Era because, post-OpenAI you can stick a fork in it. That idea is done. It is the Legacy Cloud. Still walking, not dead, but pretty dull.
Cloud 1.0 is over, because training generative AI systems requires an entirely different class of supercomputer. It is a bit old school but refreshed in the network layer. What you want now is Cloud 2.0 with a shared memory supercomputer that is transparent to manage and program.
If it is from NVIDIA, it looks like one Giant GPU. It ain't a Grandpa Google cloud.
This is what Larry Ellison and the Oracle team clocked, so they built many of them.
That is what they call the Oracle Cloud Infrastructure (OCI), or Gen2 Cloud.
The Hadoop Bubble and the Return of Scale-Up Computing
As you can well imagine, a major R&D center, like the NCSA is not short of intelligent folks. There were reasons why they focused on tightly coupled supercomputer designs. These are essential for physical simulations used in engineering digital-twin models of systems and structures, for climate system models of the Earth, for drug design, and defense.
What happened with computing in the era just completed is that Internet scale commerce had no need of such workloads. There was not the market for such high-end systems, except in a niche like nuclear weapons design, quantum chemistry for biotech, or engineering research.
Prior to the growth of hyperscale cloud computing, most such work went to firms like IBM and Microsoft, and hardware vendors like Intel, Dell and Hewlett Packard. Even in networking, the requirements were specialized, and not met by mainstream firms like Cisco. There were very different niche standards and solutions, such as Myrinet, and Infiniband, not Ethernet.
At a much deeper level, the software stack was very mathematics intensive, and required a specialized set of techniques, such as Message Passing Libraries (MPI), and a succession of oddly named software libraries, such as LINPACK, LAPACK, and ScaLAPACK.
These tools were essential for the class of high-performance computing that is important to engineers, physicists, chemists and computational biologists. Leading lights in algorithm development, such as Dr Jack Dongarra, may have been household names in traditional supercomputing, but were rarely mentioned in the scale-out era of the Internet.
In essence, none of the above mattered to Google. They honestly did not need to worry about such stuff, and did not worry about it, for a very long time. And then came Hadoop...
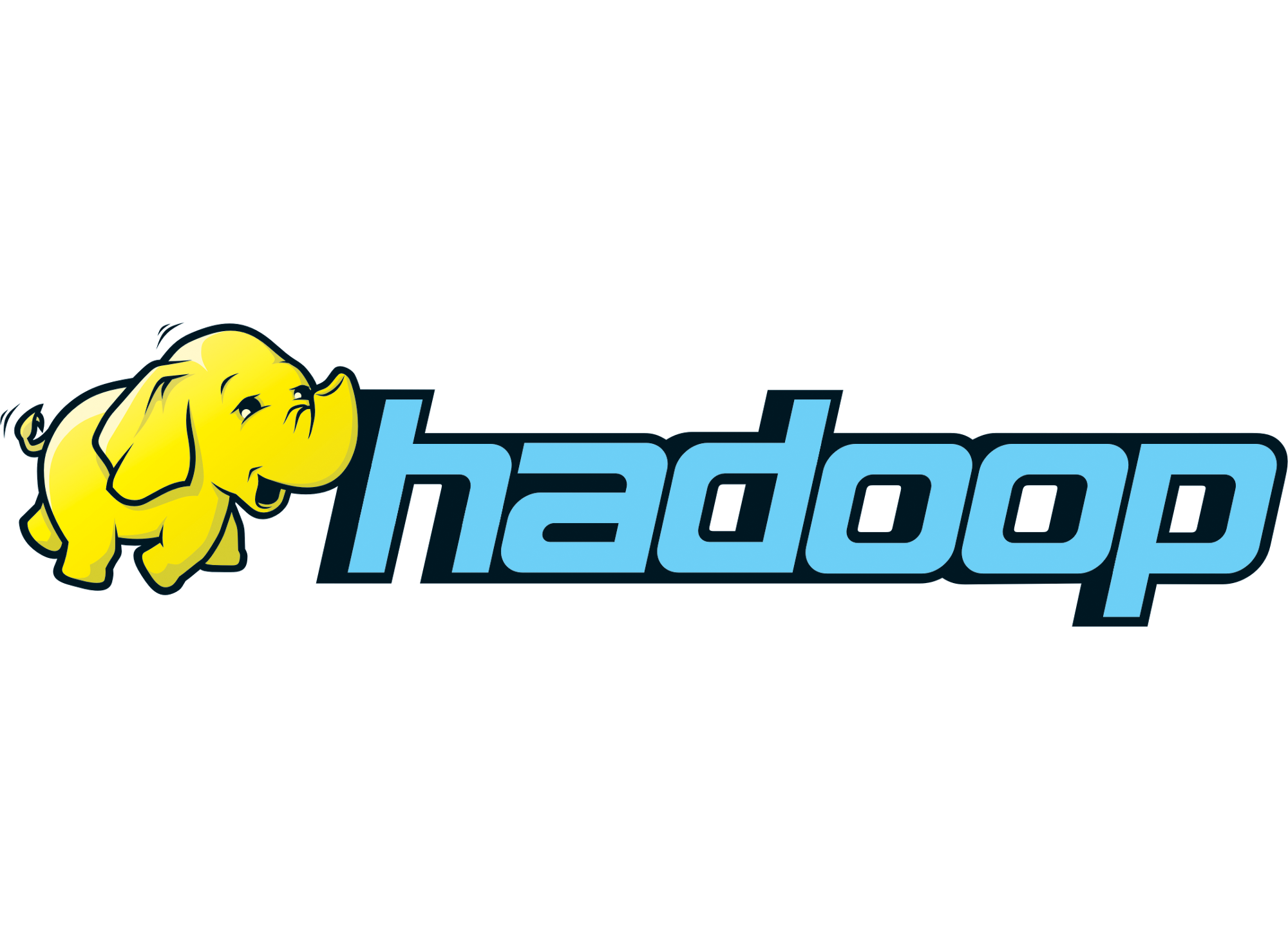
Earlier, I mentioned the Google File System, and the key role that it played in the success of Google in monetizing their Internet scale search and ad-serving solutions.
Hadoop was the first of a long line of open-source software solutions which built on this legacy and mindset to provide commodity access to data science across very large databases. While the design was innovative, and attracted a broad following, the basic ideas soon ran into a succession of roadblocks that relate to the kind of problem that you are trying to solve.
In essence, Hadoop follows a strategy called Map-Reduce, which works extremely well for the problems that Google needed to solve between 1998 and about 2018. We don't need to go into the gory details but mention that the kind of work involved is similar to creating a huge number of small tasks, describing each in an envelope, mailing that to your workers, and then collating all of the results sent back by each into one big list, which is the answer.
If that is all that you need to do, then these systems work just fine.
However, whenever you encounter a task where each worker needs to communicate with all of the other workers, to share some intermediate information, then this strategy fails. Essentially, the network becomes blocked by all the chatter between workers, as they cooperate. This all sounds very arcane. You can hear the conversation in the board room now.
Surely this AI training stuff cannot be that hard. Just do X!
Sometimes, such an attitude will work, unless the mathematics says otherwise.
The killer problem area is what are called collective operations. This is exactly how it sounds. Whenever your workforce, people or computers, needs to communicate heavily in order to complete the shared task, the progress will bottleneck, and productivity falls away.
In the corporate context, too many minds to persuade, soon leads to inaction. The same is true of high-performance computing. It does not matter if you are using a CPU, or a GPU, the moment you have many, and the need for them to cooperate, you will be writing algorithms that demand collective operations. People like Jack Dongarra, get honored with the industry equivalent of the Nobel Prize, namely the ACM 2021 Turing Award, because what they said, and what they did, through the algorithms they developed, changed the world.
Dongarra has led the world of high-performance computing through his contributions to efficient numerical algorithms for linear algebra operations, parallel computing programming mechanisms, and performance evaluation tools.
When we join the dots on this, take note of the winners of the ACM 2018 Turing Award, Yoshua Benigo, Geoffrey Hinton, and Yann LeCun. They received the same award for their algorithm developments in computing to make deep learning methods viable.
Where both of these contributions meet is in that place where Hadoop failed, as a platform for large scale data science. The low-level innovations for which Dongarra was acknowledged are the foundation for implementing the linear algebra, and optimization methods for training AI models, that were invented and refined by Benigo, Hinton and LeCun.
The place where all of this came together, in software, is the CUDA-X accelerated computing stack of NVIDIA, whose development philosophy has been led by CEO Jensen Huang.
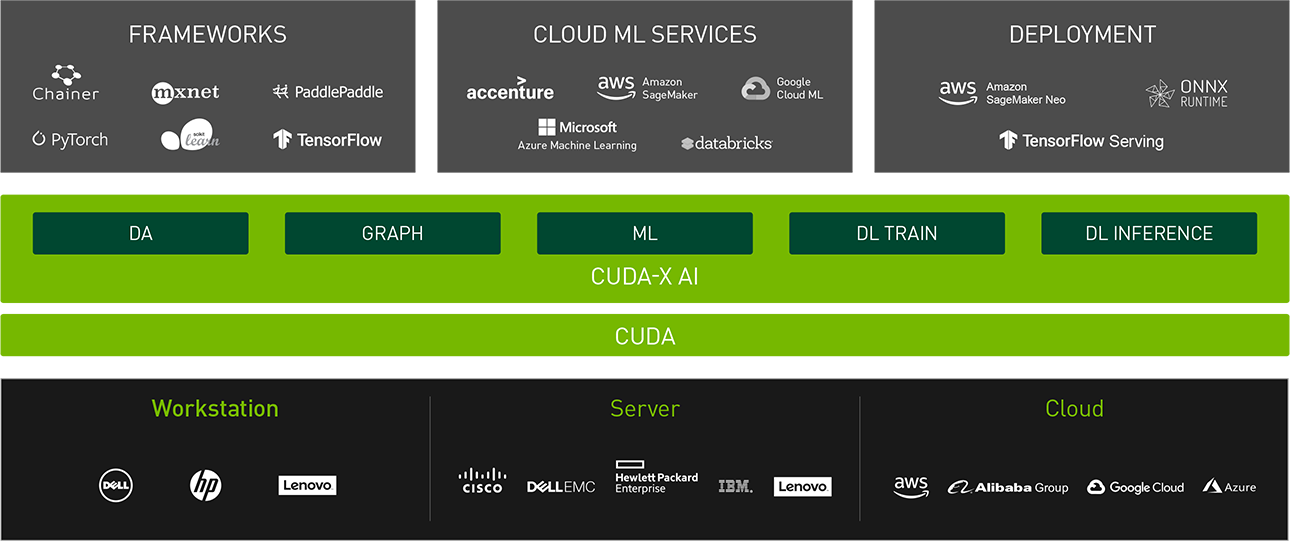
There is no great need for a deep dive on this part. What the NVIDIA stack delivers for software developers is a set of optimized math libraries. These have arcane names like cuSOLVER and cuSPARSE, and cuTENSOR, or the fetching, but mysterious, AmgX.
In among all of those, is the erstwhile, but evergreen cuBLAS.
That stands for "Basic Linear Algebra on NVIDIA GPUs." For those who don't know about this space, it is smokin' for doing math on an NVIDIA GPU. Just like Intel MKL is for x86 CPUs.
Among the three key innovations the ACM cited Jack Dongarra for, was this one:
- Batch computations: Dongarra pioneered the paradigm of breaking computations of large dense matrices, which are commonly used in simulations, modeling, and data analysis, into many computations of smaller tasks over blocks that can be calculated independently and concurrently. Based on his 2016 paper, "Performance, design, and autotuning of batched GEMM for GPUs," Dongarra led the development of the Batched BLAS Standard for such computations, and they also appear in the software libraries MAGMA and SLATE.
Arcane stuff, but that is what algorithm scientists call "Linear algebra across many GPUs."
That is the good stuff. Just what you need. It is what NVIDIA bottled up inside CUDA-X.
When we join the dots back to the Hadoop phase of data science, one may remark that what was missing, in that design, was all of that insight and knowledge bottled up in CUDA-X.
The sometimes acerbic, ACM 2014 Turing Award winner Michael Stonebraker, wrote as much, at length, over a period of years around 2010-2015. The essence of this "conversation" was a battle between proponents of "Just Scale-Out" computing (Google) versus "Just Scale-Up", which was the traditional sales pitch for a Big Iron IBM Mainframe,
The world we are now entering requires a new and more subtle mindset:
Scale-Up and Scale-Out, but don't forget that the Network is the Computer.
This is the bedrock of the accelerated computing strategy of NVIDIA.
Accelerated computing uses parallel processing to speed up work on demanding applications, from AI and data analytics to simulations and visualizations.
When you dig into the product offer from NVIDIA, it involves the CUDA-X software stack to seamlessly bond their computer hardware across both GPU and CPU architectures, with a market leading position in low latency high performance networking. Topping this is the new class of processor they invented, called a DPU. This shunts data and is pretty cool.
NVIDIA also has two related, but distinct, networking equipment offerings. One tech stack is called Spectrum-X, based on the redoubtable Ethernet, and the other is called Quantum-2, based on the little-known InfiniBand. Each is based on technology acquired from Mellanox.
It is an interesting story, and relates to the NVIDIA hardware strategy, but I won't go into the details here. The bottom line is that there are two high-performance networks out there and NVIDIA has both covered. Oracle is using the Ethernet one, which we will now explain.
Oracle and NVIDIA: The Black Magic of RDMA Networks
Let's now get down to business on why we like Oracle, in a world where NVIDIA is clearly running away with the generative artificial intelligence hardware prize. We think there is an application layer software prize on offer, and Oracle appears to be closing fast on that front.
Earlier, we explained the schism that developed between two forms of supercomputers, the Google scale, loosely coupled commodity supercomputers, and the traditional tightly bound high-performance supercomputer. These are different beasts, and the proof of the pudding rests with the need for collective operations when doing the computation.
Recall that the ACM gave Jack a gong for having helped figure out that piece of hard stuff, from the software point of view. The team led by Jensen Huang at NVIDIA, worked out the hard stuff on both the compute side, of the GPU, and the networking side, through their acquisition of Israeli-American networking firm Mellanox, which completed in 2020.
Throughout the web-scale era, the focus of NVIDIA on the networking side of computing has been largely ignored by the mainstream of the financial analyst community. In our view, this likely followed the prevailing "Google-cannot-be-wrong" mindset in the industry. There was a time when the industry mantra was "Sun-cannot-be-wrong".
They were about the opportunity that Google seized.
The entire reason I shared the above ancient history, especially the pivotal role of Sun, in the history of high-performance computing, was to make the age-old point:
Sometimes, the right way is really the wrong way. It is all horses for courses.
When we speak of hyperscale computing, it is essential to understand the application space. The so-called embarrassingly parallel problem space of mass-market web applications fits extremely well with the Google commodity-compute cloud strategy.
However, there is now a huge new class of problems facing the cresting innovation wave of massive physical and graphics simulations for digital twins, alongside generative artificial intelligence. These problem classes require collective operations. They demand the kind of mathematics library which NVIDIA has worked for 15 years to perfect, in CUDA-X.
It is this factor, more than any other, which sets NVIDIA apart from the competition, in our view. There is some magic in the choice of accelerated computing, as the marketing phrase employed by CEO Jensen Huang. The reason we investors should pay attention is simple:
All successful marketing follows a clear articulation of client benefit.
NVIDIA will do well from this trend, because they have focused on solving for the hard stuff, in software, hardware and also networking, to one single port of call, those who are expert in doing it faster, in a smaller rack footprint, with lower capex, and less power consumption.
You can see, from the NVDA share price, that this pitch is both timely and working. The stock is clearly a must own in this space, but now looks overbought in the short term.
What of Oracle? This is how CTO Larry Ellison pitched it on the call...
The hardware and software in Oracle's Gen2 cloud is fundamentally different than other hyperscaler's clouds. The CPUs and GPUs we rent to customers are interconnected using an ultra-high-performance RDMA network, plus a dedicated set of cloud control computers that manage security and data privacy.
That is the technical pitch. Of course, the RDMA networking technology is not new, it has been around a while, in other clouds. Microsoft Azure have offered RDMA-enabled virtual machines, for some time. Similarly, Amazon AWS, has offered their Elastic Fabric Adapter.
Here is Larry once again, with the money pitch:
And, in the cloud, since you pay by the minute, if you run twice as fast, and we do, then you pay half as much.
I never said what RDMA is, and nor did Larry. No need. Superfluous to the pitch.
Evidently, you don't need to know as you are destined to save money by going to the Oracle cloud and have your security and data privacy taken care of.
Needless to say, if any corporation who has heard of Oracle, and that is everybody because the reputation of the legal team is legendary, you are pretty much sold on those three points. The alternative is to go to Microsoft Azure, Amazon AWS, or Google Cloud Platform, and become lost in a baffling world of choices, with few of them marked:
Run twice as fast and pay half as much.
That is marketing, and it is what will get customers signed up for any cloud color, so long as it is Oracle orange, and comes wrapped in secret RDMA black magic cloth.
You think I am joking, but I am not really.
The hardware technology behind this AI cloud offering was worked out by NVIDIA. Oracle has done quite a bit, they have set up the data privacy and security layers, plus a network fabric management tool so that you don't have to be a career supercomputer specialist.
Oracle will be the first to offer the NVIDIA DGX AI Cloud.
There is the RDMA thingy, and that is the black magic to move the data around, effortlessly.
Oracle CEO, Safra Catz let the financials speak for themselves, on the earnings call.
Oracle’s revenue reached an all-time high of $50 billion in FY23,” said Oracle CEO, Safra Catz. “Annual revenue growth was led by our cloud applications and infrastructure businesses which grew at a combined rate of 50% in constant currency. Our infrastructure growth rate has been accelerating—with 63% growth for the full year, and 77% growth in the fourth quarter. Our cloud applications growth rate also accelerated in FY23. So, both of our two strategic cloud businesses are getting bigger—and growing faster. That bodes well for another strong year in FY24.
The important part is the infrastructure growth rate, which is the NVIDIA heavy cloud.
Customers are signing up, many of them AI start-ups like Cohere, MosaicML, and HyperReal. The reason is pretty much as Larry Ellison described; you get great technology from NVIDIA, with Oracle data privacy and security, plus a great customer service experience to get going.
This was enough for Uber to select Oracle Cloud Infrastructure and sign a seven-year deal.
The World Went Weird: Why Oracle and Why Now?
What on earth is going on?
How come Larry from Oracle is kicking goals and posting customer wins?
I think it is pretty simple. Just as Google swept away Sun Microsystems in a blaze of money saving networked commodity hardware, Oracle was the first to really step up to NVIDIA and execute a simple customer-value creating partnership deal.
We are all in with you, because your hardware solutions are the best.
That is what is really going on, and the other cloud vendors risk becoming legacy operators due to a basic confusion on who they are actually trying to serve. Are they maximizing customer benefit through renting them the best hardware money can buy? Or are they caught up with internal politicking and infighting over the "Not Invented Here Syndrome"?
I think it is the latter. There are too many internal axes to grind for Google to go "all in" offering NVIDIA, or Amazon AWS, or Microsoft Azure, for that matter. This gives Oracle a runway.
Perhaps my tone seems to make light of CEO Safra Catz, and Executive Chairman and CTO Larry Ellison. That is not my intended meaning. I think they are doing a splendid job!
I think so highly of their effort, and the runs they are posting, that I bought the stock.
I bought it off the back of a huge run-up into the earnings call.
Why not buy it sooner?
Well, that would have been sensible but, like the analysts on the call, I was a bit bewildered to think that Oracle might make a splendid comeback into the heart of enterprise computing.
That looks to be what is happening, and it is down to a secret sauce that made Oracle into a powerhouse enterprise vendor. Create a great solution, deliver value, prove value, and then ensure the customer remains sufficiently satisfied to keep paying for it.
Larry Ellison can sell a value proposition and Safra Catz can manage the firm and finances.
Oracle is back, and that would appear, against all odds, to be a fact.
Photo Credit: Oracle co-founder Larry Ellison in 2018. Photo by Bloomberg.
Never miss an insight
If you're not an existing Livewire subscriber you can sign up to get free access to investment ideas and strategies from Australia's leading investors.
And you can follow my profile to stay up to date with other wires as they're published – don't forget to give them a “like”.
3 topics
8 stocks mentioned