Nvidia needs more to go right than you think
I want to challenge some assumptions about the AI market and where big tech is looking.
Many are happy to invest in the sector without trying to understand the technology, but this can be dangerous.
I’m not trying to predict the future here, but instead, broaden your thinking about investing in AI.
You have likely heard the Nvidia versus Cisco comparisons and the threat of a dot-com-like bubble.
Let’s ignore the wider market risks for now and assume these stocks still have a clear runway ahead. I’ll cover market risks and some fresh stocks to consider in the next article.
So, what assumptions should you challenge when considering investing in Nvidia and big tech?
Assumption #1: Competition isn’t coming
Senior Strategist at Goldman Sachs, Ben Snider, called AI the ‘biggest potential long-term support for profit margins’.
But that doesn’t necessarily mean Nvidia’s…competition is coming.
Nvidia currently holds around 90% of the global AI chip market, but history and economics show this is unlikely to remain the case.
While far behind, AMD and Intel are hot on their heels with their own technological breakthroughs.
AMD’s MI300 family of data centre chips has seen strong demand recently, and Intel’s heavy spending could also shift the needle.
Intel’s latest breakthrough, ‘PowerVia’, could be a game changer in reshaping semiconductors.
By shifting power routing to the bottom of the chip, it simplifies the fab process while improving other factors. Offering boosts in performance and large energy efficiencies.
Here’s a basic image showing how that looks for transistors:
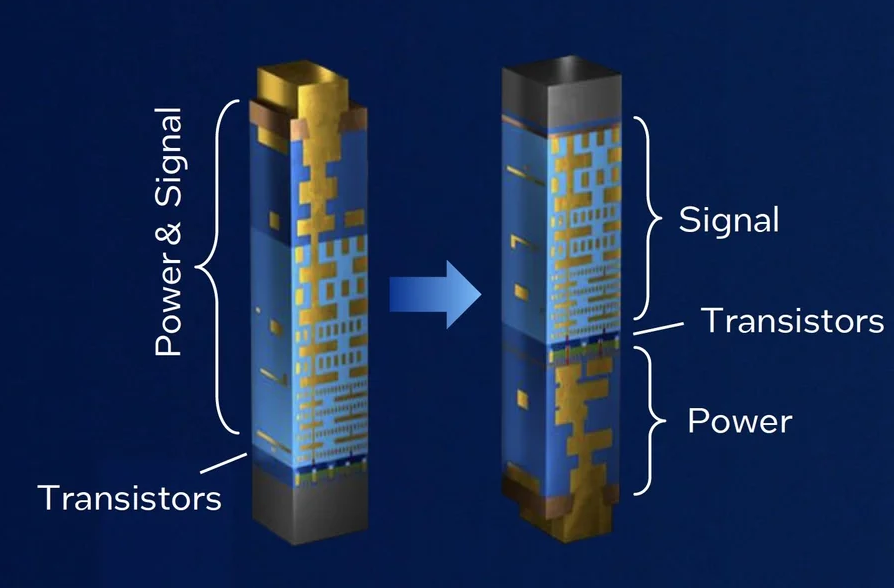
In the background, an important shift is also occurring in the semiconductor fabs.
The industry is changing from its decade-long transistor design of FinFET to the new GaaFET (Gate-all-around transistors).
This opens the door to competitors for Nvidia’s main supplier, TSMC, which will introduce its GaaFET chips later than Intel and others.
Both of these changes are important for big AI data centres, as their margins depend on low power costs and slow chip depreciation rates.
These are just two points in a tide of changes that are coming in the chip industry.
No one thing will swing market share in their favour, but a series of advances could see them improve their slice.
As more players emerge as viable alternatives to Nvidia’s AI chips, their margins will inevitably shrink.
Price competition and market saturation will mean fewer returns from their high capex spend.
Beyond the hardware, there are also changes on the software side that could disrupt Nvidia.
Assumption #2: The needs of AI aren’t going to change
Context matters. This is true whether it’s a human or AI looking at a piece of information.
The mechanism through which AI acquires contextual understanding is known as a ‘transformer’.
This is the secret sauce that turned ChatGPT into a phenomenon. AI went from a next-word guesser to a model that understood the relationships between things.
GPT literally means ‘generative pre-trained transformer’.
The breakthrough came from a 2017 paper called ‘Attention is All You Need’. The big unlock here was a mathematical way to assign relevance between words.
I am bringing up the paper for two reasons; bear with me because it will help you understand Nvidia’s market.
First, this paper and transformers are now seven years old — a lifetime in tech.
Yes, it has seen iterative improvements over time, but expecting AI to retain this architecture seems very unlikely. Why?
Transformers work by looking at how each word relates to every other word in a text.
This means that as you add more words, the number of connections the transformer needs to make grows much faster than the number of words itself.
Making longer text increasingly demanding on computer resources. Every time you double the words going in, the costs quadruple.
This becomes a bottleneck for AI chips if they want to learn relationships between distant words or data.
The big AI players have been widening this ‘context window’ or working memory to bolster AI ‘intelligence’.
That is because if it can remember more, it can reason better, remember more complex tasks, and have smarter conversations.
It’s also a clear strategy for big tech, who want to offer more voice use and team collaboration with AI.
In fact, both OpenAI and Anthropic announced major moves in this direction just last week.
OpenAI made a recent acquisition-hire deal with Multi, a video-first collaboration platform that focused on enterprise solutions. While Anthropic announced 'Claude Projects' in the same vein.
However, if they wish to continue in this direction in the long term, the transformer architecture must change. With it, chip needs could shift away from Nvidia’s specialty.
Put simply, AI software can change much faster than hardware.
The standard chip takes around two years from design to production. Software can change in months.
If a big shift occurs, sentiment around Nvidia’s stock could sour quickly.
Assumption #3: The market for AI chips isn’t going to change
Training an AI model is extremely expensive.
The last generation of models cost around US$100 million each to train, while the current generation is around US$1 billion.
Because training AI uses mountains of data, chips must take advantage of parallelism (multiple threads of work).
This was the X factor that propelled Nvidia’s GPUs from the gaming industry into AI.
It’s also why big tech companies are happy to pay huge sums for Nvidia’s GPU chips to train their latest AI.
Just look at how much of their latest earnings came from these AI data centre chips and services.
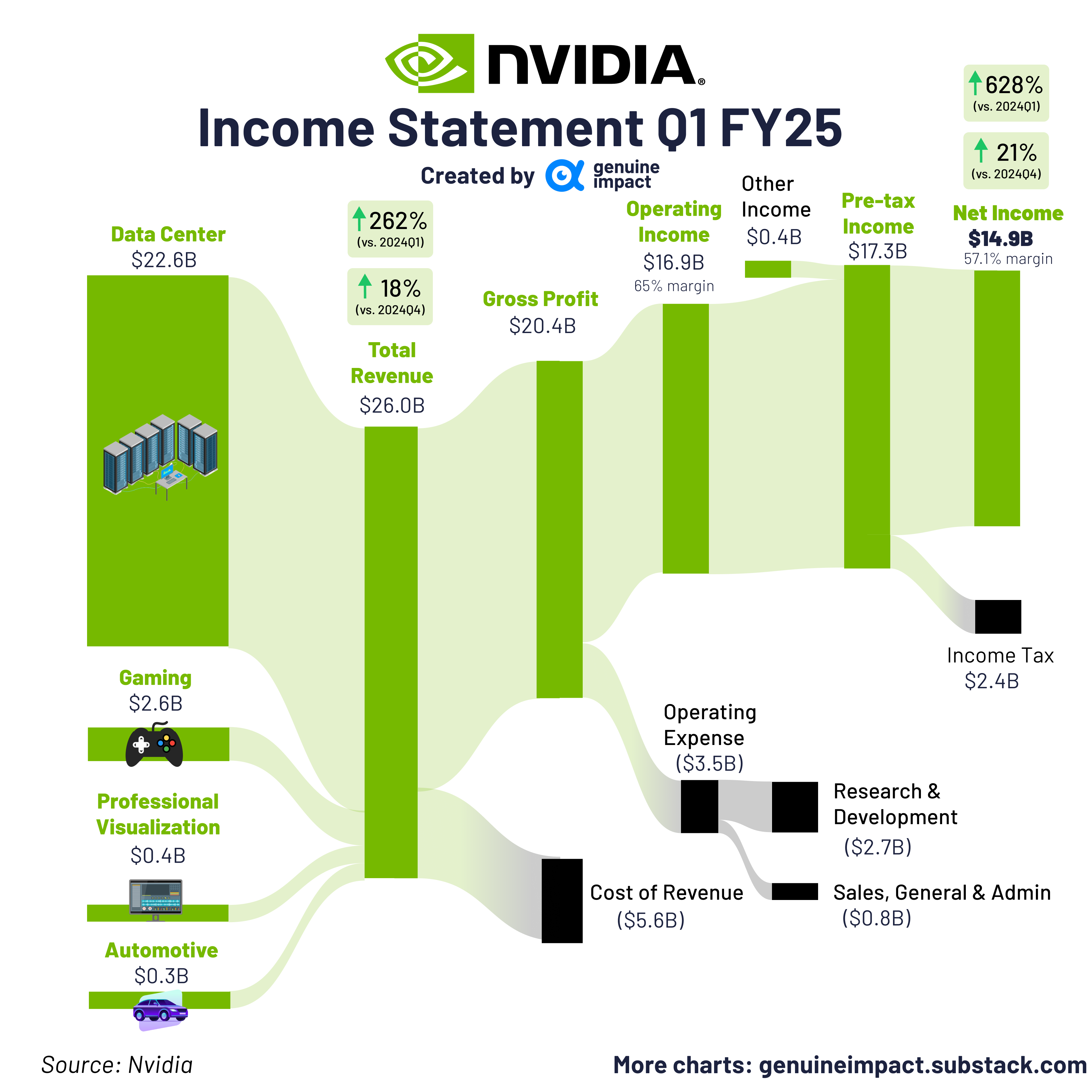
But this can almost be seen as a one-off cost. Yes, they’ll always want to train newer AI models, but companies favour chips with other qualities once they’ve trained them.
Lower energy costs are primary among them. Once you train your AI, it must serve users with inference tasks to recall that information without breaking the bank.
The market is shaping into one where big tech builds ‘foundation models’. Then, smaller companies use these to train their own AI.
That means far less training for the bulk of the market. So, once the big tech spending cycle slows, Nvidia could struggle more than others.
This major factor could split the AI chip market in two: chips to train the models and chips for inference.
A good example of this is Google, which is simply building its own chips instead of buying Nvidia’s.
A host of startups are also emerging from stealth with their own ASIC chips, which can be more focused on one task.
If they balance costs, these specialised chips could also erode Nvidia’s market share.
Ultimately, inference is a 100x market compared to training.
Nvidia knows this and has begun to move towards chips with broader applications and lower power costs, but again, competition is biting at its heels.
Assumption #4: Nvidia is the only game in town
Returning to the dot-com era comparisons, it’s important to recognise the impact network effects had in determining the winners.
Networks with growing users and data become more valuable. This then attracts developers and other services, which turns the flywheel.
Amazon and Meta are shining examples, but Nvidia is no slouch here.
Its CUDA software is an incredible offering that turns complex AI tasks into plug-and-play for GPUs. For now, it could be enough to keep developers and chip buyers in its ecosystem.
In fact, French courts have revealed that they intend to bring the first anti-trust charges on this software for its dominant position.
Beyond the courts, players like Qualcomm and AMD are playing catch-up. But other open-source alternatives could also emerge.
And if Google or Amazon can harness the power of AI with their extensive user bases, the impact could be enormous.
The big takeaway here is that, yes, Nvidia is dominant, but the path to remaining in this position is getting narrower each day.
If I were a betting man, I wouldn’t lay all my chips with Nvidia.
If you want to learn more about what the next phase of competition looks like or ASX players in this space, sign up at Fat Tail Investment Research to see more writing like this and other market-leading topics on gold, commodities, and macro trends.
3 topics
3 stocks mentioned