The Vega Way of Investing Capital
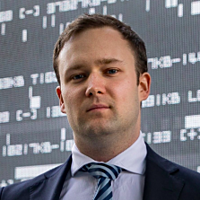
Vega Capital
Buying undervalued stocks is traditionally the way investors seek to deliver returns over the long term. At Vega Capital, we utilise a differentiated way of looking at the markets in the United States and discuss it in today’s blog.
There are two overarching algorithms we utilise, the first uses macroeconomic data to tell us whether to be biased short or long. The second identifies which investments we should enter.
Introduction
It goes without saying that we want to be long market exposure as the economy is expanding and short when the economy is contracting (i.e. going into a recession). Thus to begin, we require a methodology for understanding whether the current environment is expansionary or contractionary.
We know that the market moves from expansion to contraction and then back to expansion. This movement is what constitutes the business cycle. Ideally, we’d like to know when the contractions are about to start so we can adjust our portfolio exposure accordingly.
To do this, we utilise macroeconomic analysis via an algorithm. All data used is freely available from the US Federal Reserve.
Macroeconomic analysis
A recession is defined as a period in which GDP falls for two consecutive quarters. I’ve always found this definition to be a little strange. If US GDP contracted 1% in a quarter and then rose 2% in the next quarter, would you say this was nothing? I think most of the market would call it a short recession.
Nonetheless, we have developed an algorithm for determining when these occasions are beginning to occur.
GDP is simply production and production is the reflection of consumption. Consumption is comprised of spending via income or borrowings (i.e. consumption = spending via income + spending via debt).
The level of spending is influenced by 3 main factors;
- The money supply.
- Debt stress.
- Employment.
If there is sufficient downside pressure on these elements, a recession can emerge.
The below table shows the effectiveness of our algorithm over the past 50 years on the 5 worst recessions experienced over that timeframe. Take note of the size of the contraction in stock prices and when our algorithm gave a signal relative to the start date of each recession. Even in the cases where it was 2 months late, it ended up generating excellent results.

Let’s now look at each factor in more detail.
- Systemic liquidity (the money supply)
Systemic liquidity is a term used to describe the volume of capital in the financial system. This capital is used to make loans, provide collateral for banks and conduct general business.
It makes sense that as capital becomes scarcer, fewer loans are able to be made (and those which are made are done so at a higher price). Since a loan is made in order to finance consumption, if we contract growth of loans in the system, consumption growth must also contract – putting downside pressure on production and thus GDP.
As an addition to this, a short class in Economics 101 will tell you that as money becomes scarce, the price of other assets such as stocks should fall to restore some fair equilibrium between the two.
We can hence understand why keeping tabs on systemic liquidity would result in us understanding both where the economy is and why downside pressure may be placed on stock prices.
2. Employment levels
Given our model’s dependence on consumer and business spending, employment will obviously be a prime factor for us to monitor. An employed individual has spending power, but it also means that there’s a business which has been willing to employ her (which also implies that that business has customers who are spending).
Growing employment is bullish for an economy. More employment creates more spending (demand) which puts upward pressure on production which begets more employment and thus demand etc etc etc.
Clearly, however, when individuals become unemployed this is a particularly poor sign for an economy. Firing an employee will often cause them to stop spending which impacts the revenue of businesses and reduces the employment prospects of their employees. If the contraction in spending is sufficient, more employees will lose jobs, reduce consumption further and put other jobs at risk.
As Keynes once said ‘We’re all in this together’. And when it comes to employment, it’s certainly true.
So clearly, all this downward pressure on consumption (and thus GDP) and can cause a recession if it's significant enough.
3. Interest rate stress
If you’re a business with some debt and interest rates rise – all else equal, your ability to spend must reduce as a function of the change in rates. Hence higher interest rates can reduce consumption. The same idea applies to consumers.
(There’s one big caveat to the above, which is a wildly bullish economy in which higher rates are offset by strong consumer spending (enabled by either increasing incomes or the willingness of lenders to lend).)
Some businesses and consumers may default on their debt if rates are raised beyond their means to pay. The business may be put into administration, causing its redundant employees to stop spending (which as we’ve seen, has flow-on effects for other businesses in the economy). Consumers may not be able to take out further debt which reduces their rate of spending.
What we’re essentially looking for here is when high-interest rates become to cause problems production/consumption problems in the economy.
The Federal Reserve typically has the most control over this category for the US economy.
Checkpoint
Now that we have a set of elements which can give an early warning sign for a recession, we can determine whether our investment strategy should be biased towards long or short investments. Our next task is selecting which investments to enter.
Investment selection.
Vega Capital’s algorithm for picking option investments is quite complex and requires a fair amount of training in statistics and mathematical finance. Through some examples, however, we can communicate what our approach is, regardless of the complexity.
The below will require some basic understanding of what equity option contracts are as well as what 'writing' an option means and why options are akin to insurance.
The intuition of option pricing, losses and mispricing.
When investing in equities, investors are aiming to buy stocks below their fair value and benefit from the stock rising in price. Option investing is a little different from investing in equities.
From a philosophical point of view, we have no idea what share prices will do over the next day, month or quarter. Many investors do care about short term moves and attempt to predict them (and notably those who report to not care often do!).
We’re also less concerned with the value of the stock and more so with the value of the option. This is because even an overvalued/undervalued stock may stay overvalued/undervalued for a longer period than the duration of our option.
Writing options on stocks is very similar to writing insurance on say a car, home or other assets. We receive payment for taking on risk. Let’s explain this further with an example.
Say a standard car insurance policy is worth $1,000. This is the fair price which covers car crash expenses on a per contract basis over a group of insured drivers (ignore operating expenses for now).
If I can sell that policy for $1,500 because of high demand, then I’ve made a $500 implied profit. If competitive pressures force down the price to $800, I simply won’t write the contract because I’m likely to lose over the long haul.
How do we decide the fair price of such a contract? We might say that when we average out all crash costs with respect to the probabilities of each collision & expense, the expected claims cost per contract is $1,000. Even if I sell it for $1,500, this claims estimate doesn't change.
We do something similar in markets with option prices. The fair price of an option is the probability-weighted set of potential claims (i.e. losses) using a statistical model which encapsulates the fat-tailed nature of the market. The fair price is the value of the option.
We then consider the market prices of options on stocks relative to their valuation. As such, if we find an option valued at $10, we’d likely be happy to write it at $12 since that’s $2 of free money.
Philosophy on making money with an option investing strategy
Even if the option value is $10 and it’s trading for $12 it doesn’t mean that we’ll realise $2 in profit this time around. Let’s say an adverse stock price event transpires which has us paying out a $20 claim. We have received $12 to help cover losses, but that’s still an $8 net loss.
Of course, if we continue to write the option for $12, eventually we will realise our $2 profit per trade since the average loss will eventually converge to $10.
In the prior example, we assume we knew nothing about what prices might do next. We assumed that we were writing the option blind and that the premium will cover all eventual claims plus deliver a profit.
However, we do have some hints about what direction we should bias our option investing to i.e. our macroeconomic algorithm gives us a good idea as to where the long term market trend is going.
For example, if the algorithm says that the economy is expanding then market prices will typically be rising. So we would enter option investments that are profitable when this view plays out. Likewise, if the algorithm flags recession risk, we would look for option investments which are profitable when markets fall rapidly.
It won’t tell us every small up and down market motion, but as long as we’re with the economic trend for the most part and dodge some of the bigger declines, we should do just fine.
Coin toss
So how do we describe what this looks like all together from an investment point of view? One analogy we can use is a game of coin toss.
Let’s say that by using a unique algorithm, we can predict the outcome of the coin toss with 55% accuracy. For each heads, you win $12 and each loss is $10. The expected win per game is thus $2.10 ($12 x 55% -$10 x 45%). Hence our expected win over 20 games should be $42 ($2.10 x 20).
We've simulated this game 6 times in the below graph.

The profit and loss curve is volatile but due to the positive expectancy, an enterprising investor would be willing to invest in it as many times as possible. In the short term, it doesn’t mean they’re guaranteed a profit but over the long term, the math works out.
A few observations:
- Over the first five iterations, simulations 2, 3 and 5 are all showing a negative account balance.
- By iteration 10, simulations 2, 3 & 5 are still negative. The average profit should be around $21 (10 x $2.10) but the realised average profit is well below that at a $1 loss.
- By simulation 20, again all but simulation 2 & 5 are in positive territory. Average profits across all simulations have risen to $35, below the expectation but still quite good.
Hence from such an algorithm, we should expect considerable short term variability but long term positive expectancy….assuming we consistently stay in the game through all up and down periods.
This coin toss analogy also works for markets since the same variables apply, i.e;
- The probability of winning/losing and having a means of identifying a probabilistic edge.
- The amount of premium we receive versus our losses as well as our patience in waiting for the right opportunities to come around (i.e. we don't invest when there are no opportunities).
The outcomes aren’t unlike what you might find when investing in options. Of course, we do employ a lot of addition portfolio volatility controls (so as not to end up with large drawdowns like in simulations 2 & 5), but rather than focusing on short term returns, we focus on running our algorithms over an entire business cycle so as to maximise the probability of making strong long term returns.
What we’re seeing now.
Option prices were expensive in December of 2018. Options then became ridiculously cheap late January 2019 through March and have only now begun to show a few opportunities.
As such, we wrote a lot of options in December but have found few this year to date. We’re happy to remain patient and wait for better opportunities to arise regardless of where markets might go in the interim. This means we’ve carried the risk of underperformance versus the market in the short term, but we’re better off for it in the long term. To do otherwise would be like selling a $1,000 car insurance policy for $800 or $1,000, either too cheap or not worth it.
On the macroeconomic front, as we discussed this month with our investors, Vega’s model is estimating the probability of a recession in the next 12 months as ‘Moderate’. There are now many confirmatory signals that the economy is in the later stages of the business cycle.
These include but are not limited to;
- Bottoming out of unemployment trends (recession usually occurs 3-18 months after).
- Yield curve inversion (recession usually occurs 3-30 months after).
- Monetary tightening by the US Federal Reserve (variable recession timing depending on Fed policy).
There are however no immediate triggers for going short. It’s certainly possible that the economy may weaken early and cause us to short in the next month or two. But for now, our expectation is for a weakening of economic data through 2H2019/1H2020.
If any of the above is difficult to understand, please feel free to ask any questions in the comments below.
4 topics
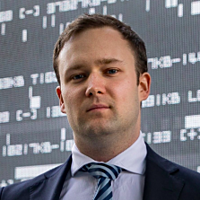
Scott has seven years of experience in investment and risk management, was previously an analyst at Montgomery Investment Management, and holds a degree Economics from the UWA as well as a Master’s degree in Financial Mathematics from UNSW.
Expertise
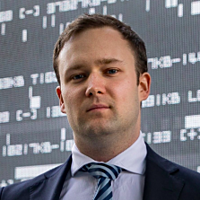
Scott has seven years of experience in investment and risk management, was previously an analyst at Montgomery Investment Management, and holds a degree Economics from the UWA as well as a Master’s degree in Financial Mathematics from UNSW.