War Lab puts risk of China/Taiwan conflict at 74% in 2020, which Ray Dalio's research seeks to emulate
Amidst news that the US has sensationally relocated one-quarter of its entire strategic B2 stealth bomber fleet to Australia due to the rising risks of war with China over Taiwan, it is worth revising our contrarian predictions on this subject.
In October last year we released pioneering academic research that for the first time (to our knowledge) quantified the empirical probabilities of different types of military conflicts occurring between two nation states using advanced statistical methods and up to 160 years of data covering a multiplicity of conflict drivers. We published this research via our interactive War Lab, on the academic SSRN website, and provided a non-technical summary through the Australian Strategic Policy Institute.
Our models, which included machine learning methods that had out-of-sample explanatory power, put the empirical probability of a China/Taiwan kinetic military conflict, including the declaration of war, at a very high 74%. They further estimated the probability of a US/China kinetic conflict at a high 45%, which likewise encompasses the declaration of war (see below). Importantly, these assessed probabilities were limited to rich military, conflict, geographical, political, economic and social data over the 160 years up to 2020: they did not have the benefit of all the new data/information that has emerged over 2020, 2021, and 2022, and which implies much higher conflict risks again. The Australian newspaper provided an excellent summary of our research here.
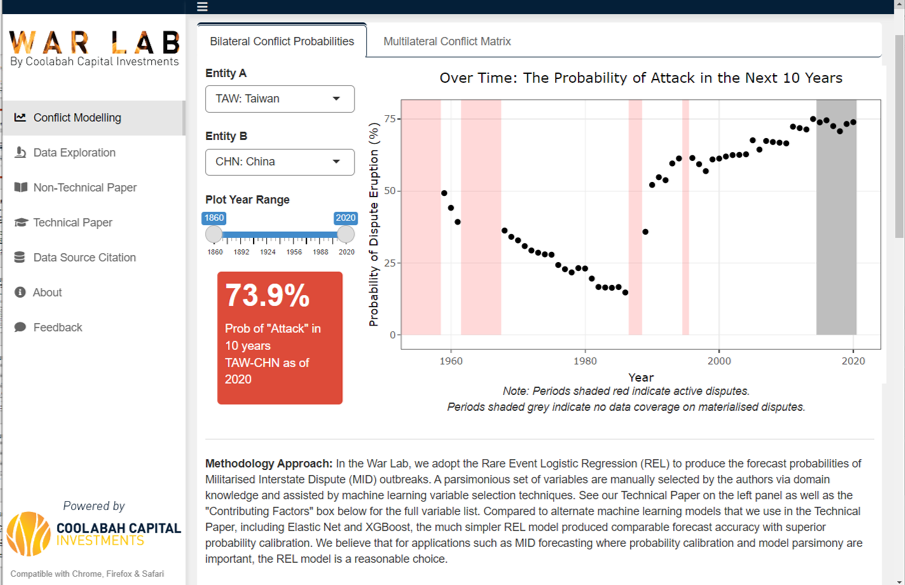
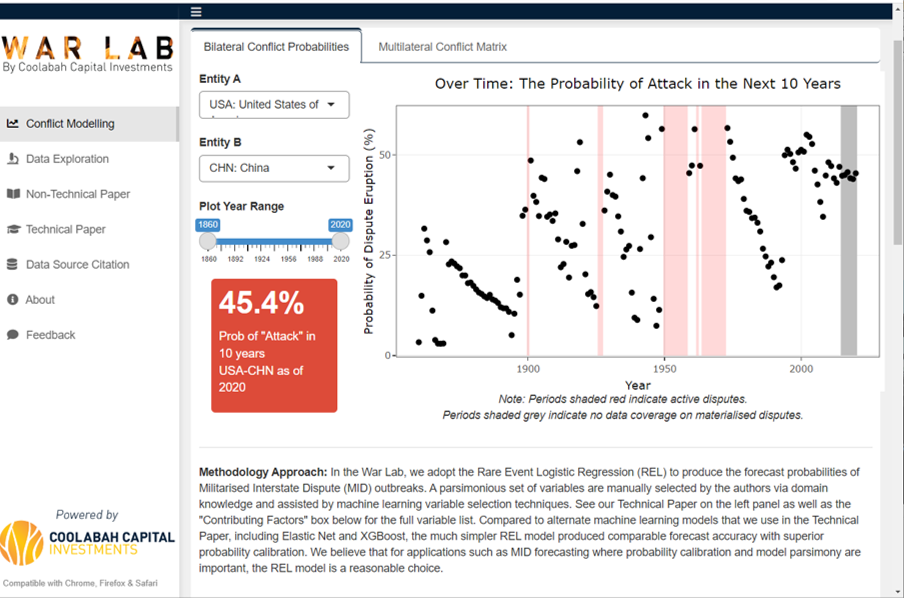
Bridgewater's famous founder, Ray Dalio, has recently released a much more basic “conflict gauge”, which is an index built from “indicators such as changes in military spending, personnel, and deployment; sentiment of each country's people about the other country; media attention given to the conflict, etc”. On August 9, Dalio highlighted the increase in his US-China conflict gauge, which has particularly popped since 2020:
The chart below shows my US-China conflict gauge since 2000. As you can see, the readings for conflict between the US and China are the highest ever.
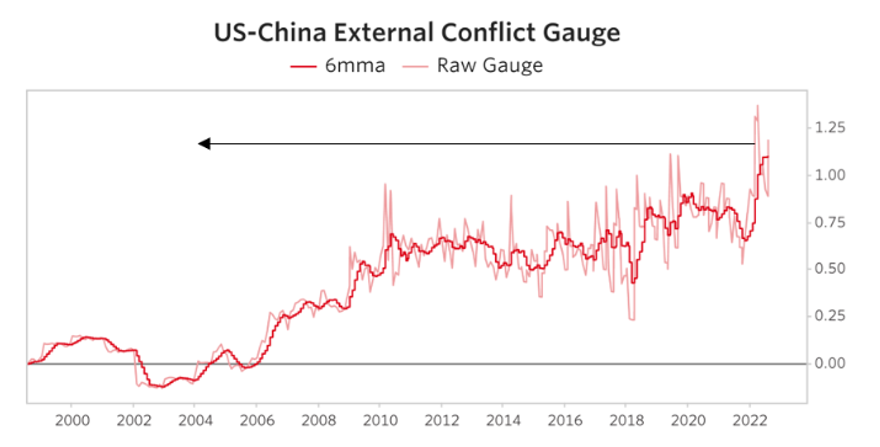
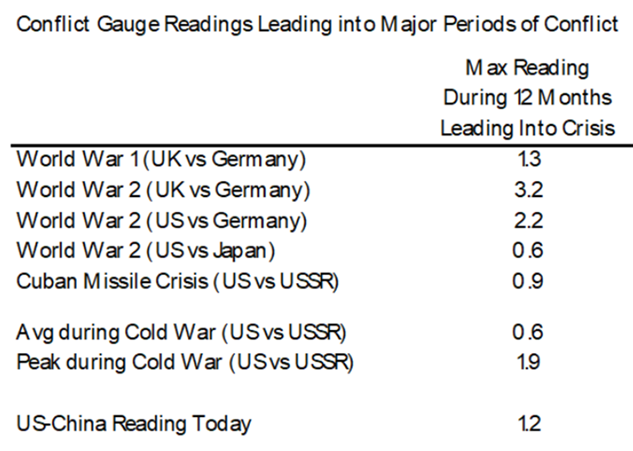
We very much applaud Dalio for seeking to furnish quantitative insights on conflict risks, which has been something of a lacuna in the global research literature. As we wrote in our technical academic paper on the subject last year:
To the best of our knowledge, the core contribution of this work to the academic literature and society more generally is the integration of a wide range of historical data sets, including predictors of militarised interstate disputes (MIDs) and advanced variable selection methods, with sophisticated modelling techniques to address a well-defined set of forecasting problems based on differing MID severities and time horizons, culminating in the generation of calibrated and accurate forecast probabilities of different types of MID outbreaks between any state pair of interest across time. We believe this is also the first time these insights and forecast probabilities have been disseminated via a publicly accessible graphical user interface system.
In contrast to our work, Dalio’s “conflict gauge” does not seek to provide an array of differing conflict-type probabilities over a range of forecast horizons for every country globally using sophisticated statistical methods. His goal is to simply develop an indicator to track the time-series change in conflict signals. The first chart below highlights some of the out-of-sample testing we completed on the accuracy of our conflict probabilities for different severity thresholds over various time horizons. Since the outbreak of the Russia/Ukraine conflict, we have been sometimes asked what our system suggested about that contingency. Back in 2020, the system had a relatively high 1-in-4 to 1-in-5 probability of a Russia/Ukraine conflict over the next 10 years, although it was severely limited by the fact that Ukraine is a relatively new country established in 1990 following the collapse of the Soviet Union. This means time-series data on Ukraine for the purposes of quantitative modelling is extremely limited in comparison to most other countries, such as the US, China, and Taiwan.
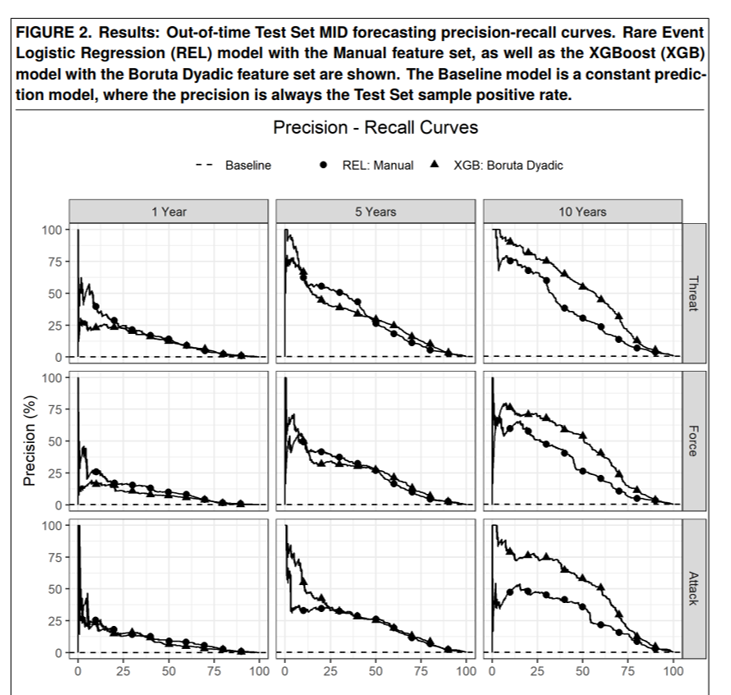
Coolabah's War Lab has a range of novel data-science tools that we developed to help the public better understanding the evolution of conflict risks, including
- a break-down of the specific variables that contribute to particular conflict probabilities (first image),
- interactive network graphs that allow you to explore linkages between different attributes of states' power (eg, military spending), political character (eg, democracies vs autocracies), different types of conflicts, and time (second image),
- and historical animations of power projection capabilities over the last couple of centuries via Voronoi diagrams, amongst much, much more.
In future research we will look to update our War Lab to bring the data-set forward from 2020 to 2022...
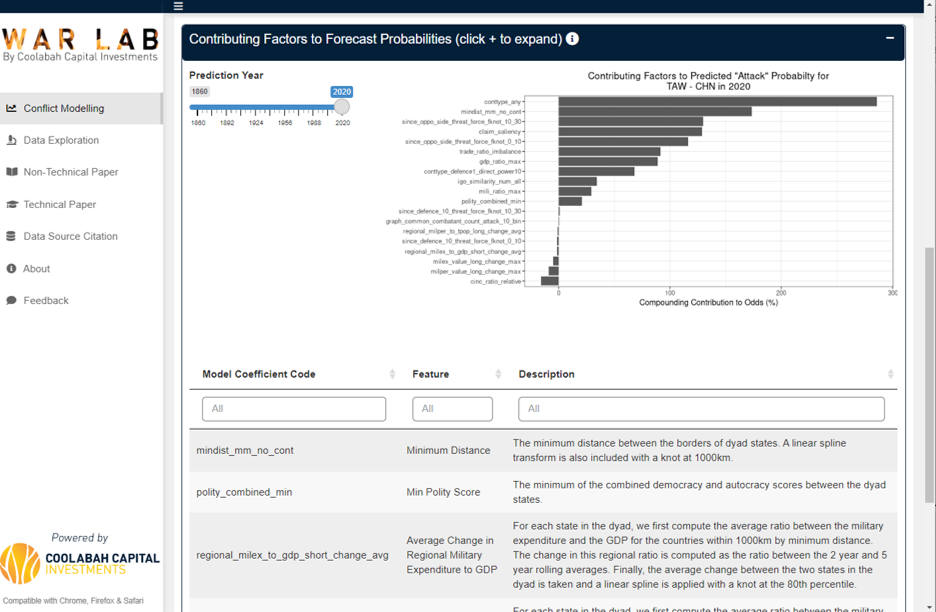
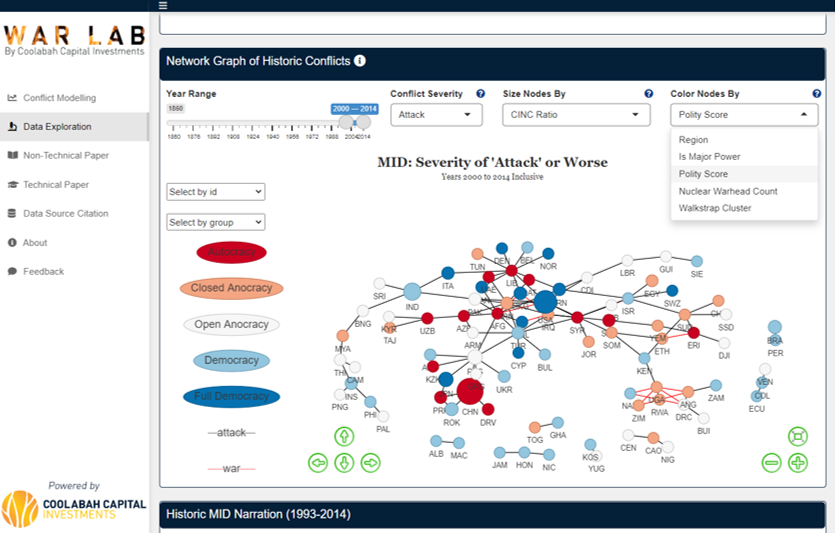
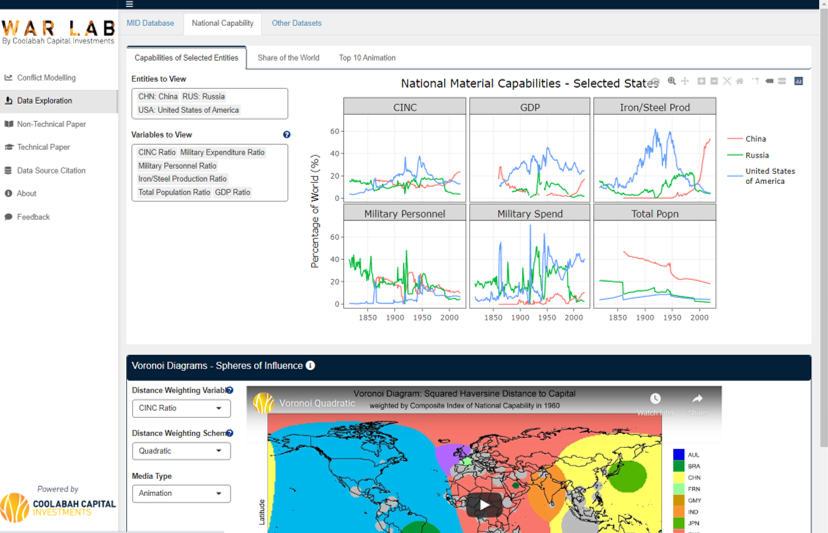
3 topics